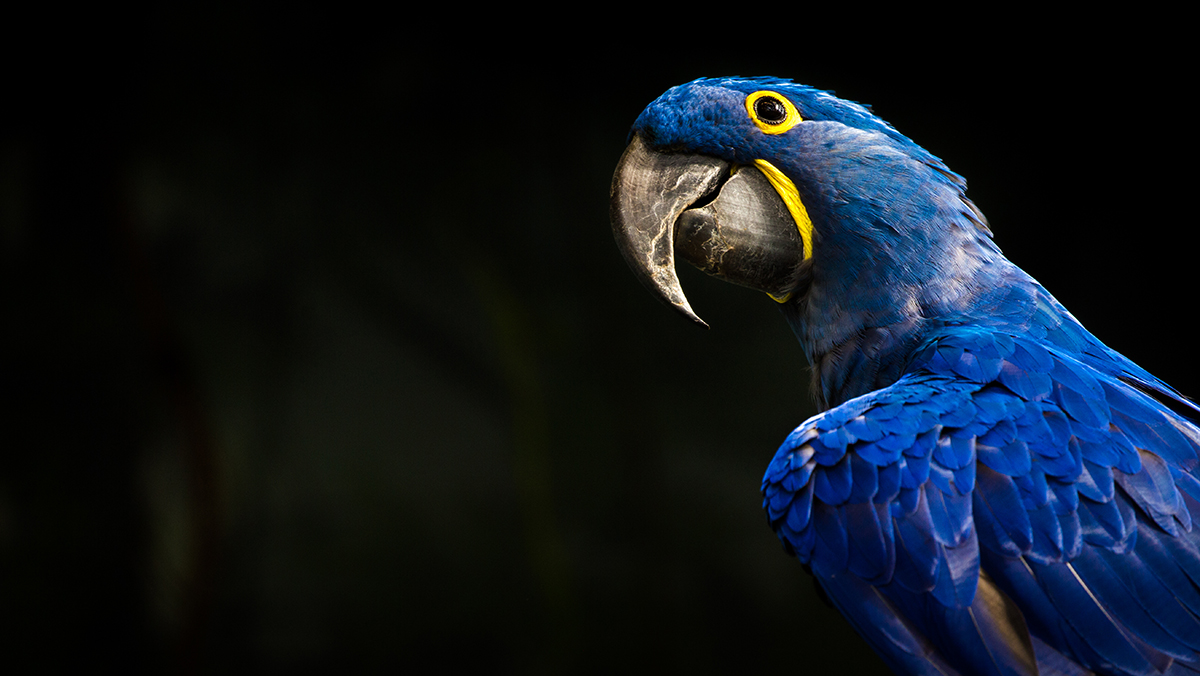
Recently one of the “gods” of AI – Yann LeCun, head of AI at Meta, who kept the faith in the dark years of neural network theory gave a talk about “Large Language Models” (LLM’s) – the foundational model at the base of GPT and its ilk. He repeated his often repeated maxim that LLM’s are just “stochastic parrots”. They are in theory simple processes that predict the next word in a sentence based on an exhaustive analysis of text. LeCun estimated that for a person reading 8 hours a day, it would take 22,000 years for them to read all the text that GPT 4.0 has been trained on.
GPT-4 is a very, very complicated statistical model of text, nothing more. It does not think. It has no consciousness, it has no knowledge of the form that humans understand. It parrots back what it was trained on, it’s just a very “pretty polly”.
At first look it seems incredible that a statistical model of language can produce the results we see but it is worth remembering that GPT is a very, very large collection of “weights”, floating point numbers, with some complex systems surrounding it. It’s simple in principle but not really in practice. GPT-4 has been trained on 45 terabytes of data and it’s estimated it has 100 trillion parameters, in simple terms a parameter is a “weight” or number used to generate the output. GPT is not small and it’s not simple.
It would seem, then, that the question of epistemology and GPT is an oxymoron, GPT is not a conscious thing and therefore, in our terms, has no knowledge. LeCun then goes on to talk about what a more complex system that could be closer to human cognition and behaviour might look like. There are many more “elements” required of such a system and a GPT like structure only fulfills one of many needs for such a system. He likens GPT-4 to “at best approximating the functions of Wernicke and Broca’s areas of the brain”. These are two areas of the brain that are well established as processing speech.
Speak, demand, we’ll answer
In Shakespeare’s Macbeth the three witches challenge Macbeth to ask him questions about what they know of his fate and how they know it. They communicate in speech, they answer with speech, their knowledge of his fate is sent via speech. We’ve all heard the saying “a picture is worth a thousand words”, which in some contexts may be true but mostly we send knowledge via speech. Books, websites, pretty much all of our knowledge is encoded in speech.
Skills, like catching a ball, can be described in speech but can’t totally be learned that way – that is a different form of knowledge, motor knowledge.
If you send an image to one of the numerous image analysis services available on the web you get back text. Not more images, but text. The dirty secret of image analysis is that a lot of it reduces an image to text, labels of objects in the image. It doesn’t send back images. (What happened to the “thousand words” idea?) You get back text.
This is because we use language almost exclusively to transmit information, knowledge and feelings. We don’t walk around carrying decks of cards with pictures on them to show people what we want to tell them. We didn’t evolve LCD screens instead of vocal cords, and we learned to write.
Exit turing
For many decades the litmus test of “is a system intelligent” was the so-called “Turing Test”. Devised by the mathematician Alan Turning it stated that if a person could not tell the difference between their interaction with a computer with that of a person, then the computer system would be deemed to be intelligent. No one talks much about the Turing test anymore, given that GPT has passed a USA legal bar exam and answered a lot of medical questions very accurately.
GPT is not thinking, but it can produce the answers. It has, in a sense, a prodigious memory that means it can guess very accurately what the answer to a question might be. It doesn’t “know” that answer by a chain of logical reasoning (it’s a stochastic parrot) but it “guesses” based on what it has been trained on.
It’s this guessing that is both the promise and the problem for brand research.
One and two
In LeCun’s talk, mentioned earlier, he references Kahneman’s theory of system one thought and system two thought. System one thought is the quick “heuristic”, guessing mode of cognition, system two is the deeper, more logical form of cognition. LeCunn says that systems like GPT can be thought of as models of system one thought. It’s all based on guessing – of a form. GPT has no way to analyse a question, it literally makes a guess as to the answer based on its past experience.
Like a consumer when they need to decide something quickly, GPT takes an informed guess.
It doesn’t seem too illogical to say that GPT has a form of knowledge that is derived from language and the patterns of language we use. Knowledge has many forms: the ability to catch a ball, how to ride a bike, how to write, how to program a computer, how to draw a realistic picture of a face. GPT has a form of statistical knowledge of language. This means it can guess at answers and it can be pretty good at getting the right answer.
So in that way, GPT’s guessing ability could be seen as a sort of system one simulation of a person. Maybe even a consumer. A paper published last year made clear that the low cost of this approach and the speed of the results make it very appealing.
Walk like a duck, talk like a duck…
If you spend any time using chatGPT version 4 you will begin to realize it is incredibly useful. I estimate my use of google search has dropped by 50% since I began to use it and related models such as Bing Chat. Moreover it can be used to simulate consumers to build hypotheses. For example, you can ask it to simulate a certain type of person and then ask what their behaviour would be i.e what would they think of a certain shampoo. Some of the results might seem obvious: a middle aged midwestern man has a different set of opinions than a 25 year old woman living in New York.
But is it obvious? How does a mere stochastic parrot produce such a result?
And systems like GPT can be “fine tuned”. You can feed them, let’s say, all the research results on a product for the last 5 years and then ask it questions about the product. You can ask it to interpret tabular data. I know that one of the largest market research companies in the world is already testing a GPT type system that analyzes tabular data. And as an added extra GPT-4 is not bad at summarizing text, which can be quite useful. Overall, it’s very hard not to think that GPT type systems are a simulation of Kahneman type system one processing.
Walk like a duck, talk like a duck… you’re a duck.
The weather forecast
I’ve always been very taken by a quote from a poem by Louise MacNeice.
“The glass is falling hour by hour, the glass will fall forever,
But if you break the bloody glass you won’t hold up the weather.”
The “glass” is a barometer, when it was “falling” it meant low pressure and bad weather was coming. As MacNeice says even if you break the glass, the weather still comes. Ignoring what is happening with GPT-like systems is pointless; they have arrived, more are coming, and they will have (and are having) a profound effect on our society.
As to the epistemology of GPT-4, clearly it has some knowledge, but not in the way we recognize as humans. It has stochastic language knowledge and this may well be a good model of Kahneman’s system one thinking. This could be extremely useful for brand strategy because isn’t that the precise mode of human thinking – the notional, heuristic response – that most brands aspire to trigger?
It’s also worth remembering that this is just the beginning; new models and technical advances are flooding out every day. And it’s also worth remembering that the so-called Luddites were not against machinery per se, indeed many were highly skilled machine operators – they just wanted this technology ethically used to make quality goods using well-qualified people.
LeCun: “There’s something like 12 million shops that advertise on Facebook, and most of them are mom and pop shops, and they just don’t have the resources to design a new, nicely designed ad. So for them, generative art could help a lot.”
Therefore, in a sense, Luddites are what we should become. We shouldn’t be using this technology blindly or haphazardly – there will be (and are already) many charlatans out there offering slapdash AI-only solutions that may, if left unchecked, discredit the entire marketing sector.
What we do need to do is engage with this new knowledge form, intelligently, creatively and above all ethically, with the all-important overlay of human inspiration – or suffer the consequences.
Cover image source: jurra8