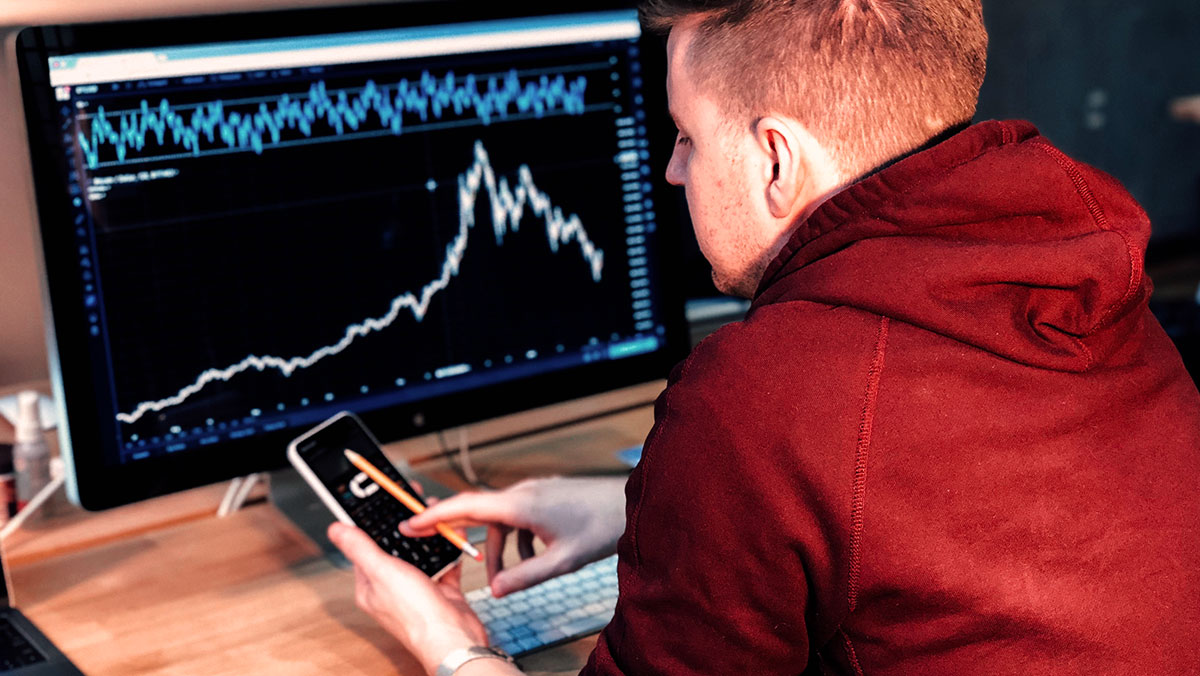
The data-driven economy has emerged in recent years. The digital revolution, the increase of connectivity, and the instant spread of information are revolutionizing the way we operate and work at the individual, company, and institutional levels. There is increasing recognition of the importance of data and their analysis through advanced statistical models and machine learning techniques. The definition of an adequate decision-making model based on relevant and actionable insights produced by advanced analytics algorithms is getting increasingly crucial for companies. The balance between the predictive ability of statistical and machine learning techniques and the expertise of management is a fundamental requirement today to drive the decision-making process and gain a competitive advantage.
Humanity is creating growing quantities of data and leaves enormous digital trails of its tastes, needs, consumption, activities, and interests. So we speak more and more of artificial intelligence (AI) and machine learning (ML). The new systems of data analysis are provoking a large change in the way managerial decisions are made. A growing number of both small and large businesses recognize the potential residing in the data at their disposal. Discovering and predicting trends, patterns, and behavior in advance is a key success factor for competitive advantage.
Machine learning to support strategic decision-making processes within companies
From an institutional point of view as well, for some years now, there has been recognition of the potential impact of the new methods of analysis. New types of skills are becoming essential: In particular, professionals able to meet the challenges of data science are needed who know how to transform the information produced by calculators and advanced analytics algorithms into indications relevant for decision-makers, institutions, and businesses. Some critical reflections on the revolution underway are necessary. The first is positive: The fact that increasingly reliable data and predictions are used to make decisions cannot but lead to an improvement in the decision-making process. The support of a theory or a quantitative formulation provides indispensable support for the weakness of the human mind at the moment it must make difficult decisions.
On the other hand, though, the danger is that of leaving decisions to be made automatically by machines without human supervision or intervention. In fact, an advanced analytics algorithm blindly follows preset rules dictated by lines of code that, in any case, represent an abstraction of the problem and cannot take into account all of the possible combinations and all of the peculiarities of specific situations. Think of an algorithm that is able to grant or deny a loan. If we were to use the following as a simplified rule of the algorithm: “If the person has been unable to pay back a loan in past years, they shall not have the right to a new loan”, then all the persons who, even only to a small extent, did not make a payment in previous years will not have access to a new loan for an indefinite period of time, even if their financial situation has clearly improved in the meantime. Therefore, we would have an unjust, but automatic denial of the right to loan access. The question is, in the presence of an algorithm that analyzes thousands of data points and produces a signal of “no, we will not grant the loan”, how can a human being, based on specific sensations, reverse that analysis? Is a human being able to assume this responsibility?
The potential of data science and machine learning on business
Prediction models – and, in particular, those based on historical statistics – have been (and, in the coming months, will be) strongly affected by the discontinuity in those statistics, suggesting the need to incorporate more qualitative research tools that consider the scenario of demand behavior. In these months of great difficulty, companies are asked to be more efficient and have a greater ability to exploit the potential of machine learning applications, with effective solutions able to concretely assist business decision-making processes.
It should be stressed that the importance of the interpretability aspects of models is not uniform in its different applications. If we think of an algorithm that recognizes images or speech or also in some tasks such as playing Go, the key point is that performance and interpretability are minor goals. But there are other applications in which it is inevitable to seek a balance between interpretability and predictive capacity, and the majority of managerial applications certainly fall into this category. For example, think of the problems linked to the propensity to purchase a specific product by a customer, the issue of customer loyalty (churn/attrition), or that of the behavioral segmentation of customers. All of these applications require the analyst to be able to provide an interpretation of results both to communicate them to management and to be able to implement the most appropriate actions to reach the goal of improving the business conditions, that are the premise of the very idea of applying a machine learning model.
Moreover, the interpretability of a model can also reveal its weaknesses, for example providing an explanation of why it fails or even showing that the decision suggested is mathematically correct, but based on imprecise or misleading data. Being fully aware of the weaknesses of an algorithm is the first step towards improving it.
The data-driven decision-making process
We thus come to the modern decision-making process. The process has been studied for a long time in the literature. In figure 1, the main steps are illustrated.
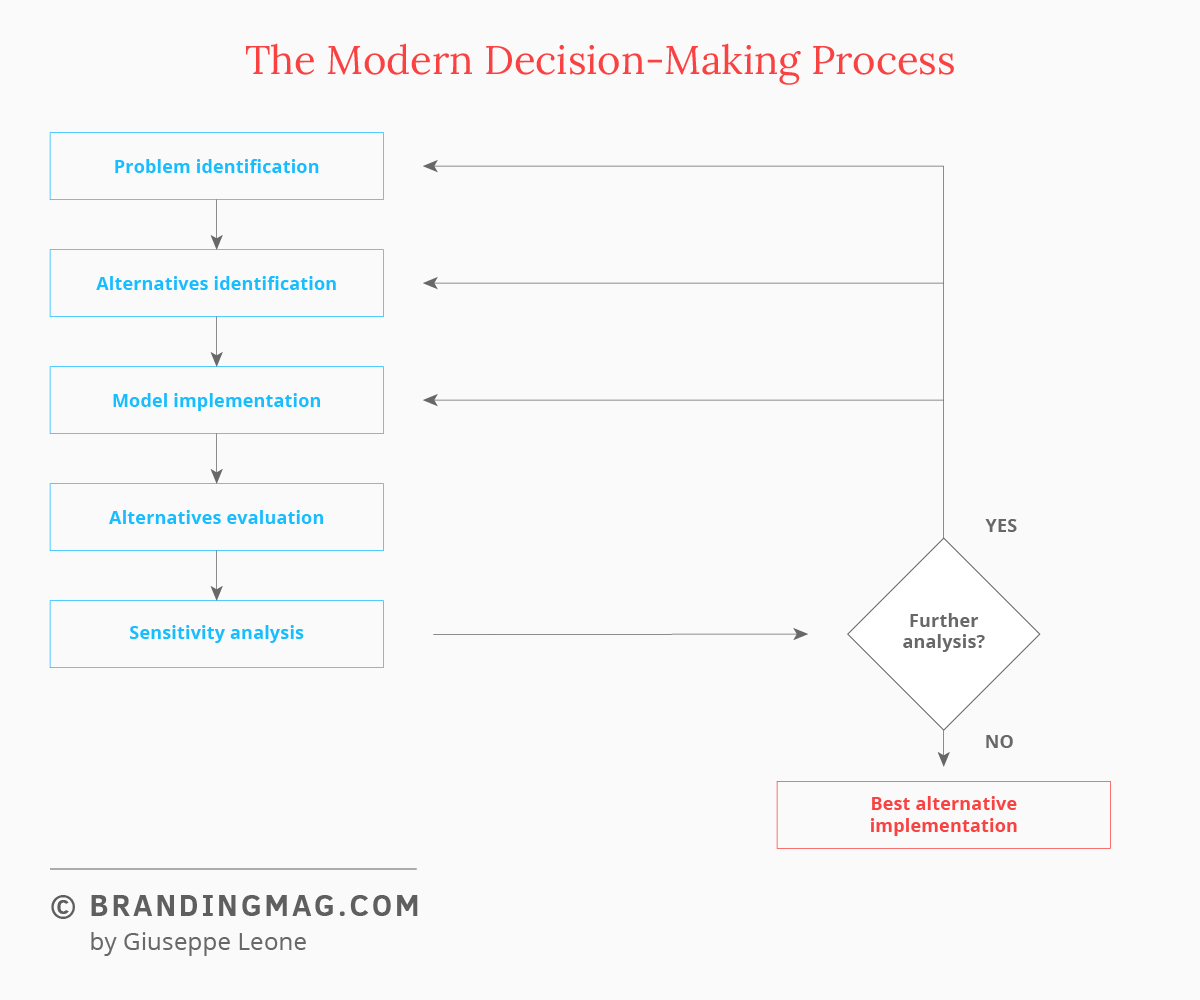
The first phase is that of identifying the problem. For example, a company that wants to better understand the brand positioning on the market or could be interested in performing a customer churn analysis. Among the available alternatives, we can consider that of performing it in-house through the company’s own personnel or entrusting the analysis to an outside supplier. At equal time and financial resources, the first option depends on the decisive condition of having the necessary internal skills to do it.
The next step is to formulate a model. This is the sum of the predictive algorithm and the available data on which to carry out those predictions. We are entering a complex world here, that of the preparation of the data and the selection of the predictive algorithm. For example, let us consider a churn analysis. In this case, the analyst could choose to opt for a logistic regression model, a decision tree classification model, a random forest, or a gradient boosting model. But it is clear that not all of the variables make sense for the problem in question. Once the ones pertinent to the problem are identified, it is necessary to then collect quantitative information on them, orienting the data collection.
Once the model is implemented, the results obtained will be examined. From the analysis of the model’s sensitivity and strength, and a careful examination of the process that has taken place to that time, it will be possible to determine if the results are satisfactory. In this case, we can move to the implementation phase. Otherwise, we return to repeat and refine some of the previous activities so as to arrive at the most robust decision possible.
The development of the discipline is such that many companies are creating internal data-science teams. This choice has the advantage of allowing the company to internalize the necessary skills, enriching the company’s human resources in a critical function for providing support to management. It also has the advantage of managing data internally, which on the one hand contributes to minimizing the risks linked to the question of privacy, and on the other allows for constant and direct monitoring.
Obviously, the creation of a data science team also entails costs associated with human and IT resources (computation and storage), that need to be entirely covered by the company and are constant in time. On the flip side, if the size of the company or the investment possibilities is relatively limited, the most flexible and practicable solution is that of outsourcing the data management and/or analysis process through the use of qualified suppliers.
Cover image source: Chris Liverani