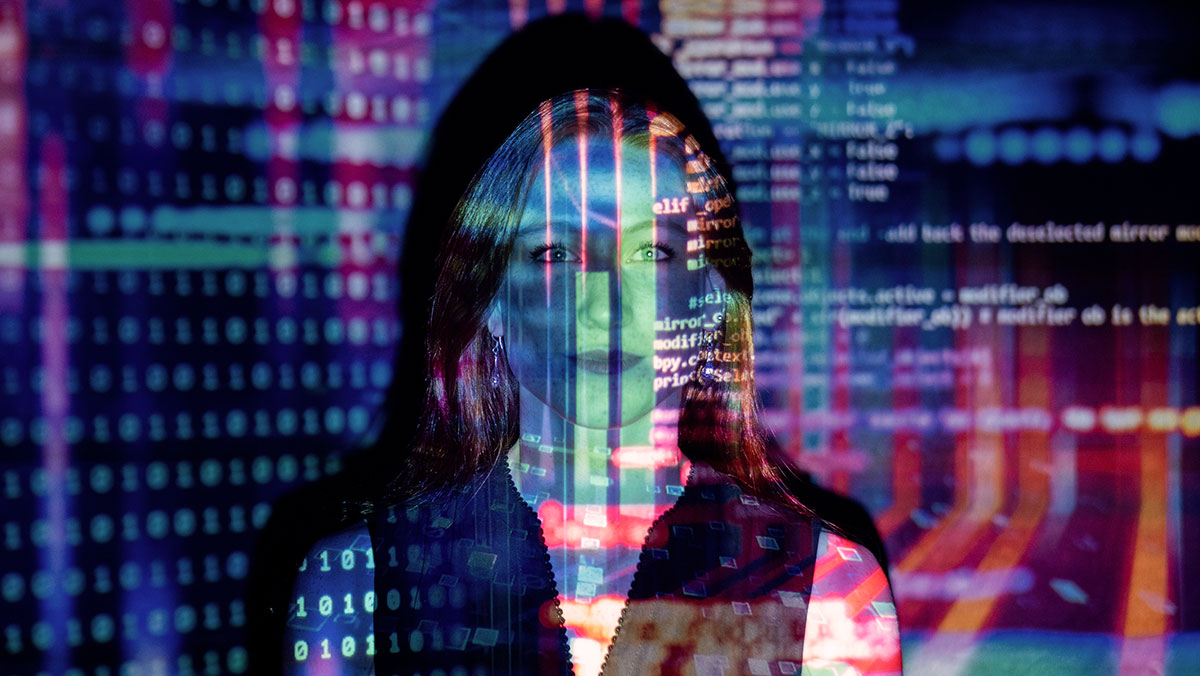
It is said that we live in the so-called “data-driven economy”, which is an economy where data is the fundamental resource for generating a competitive advantage. To understand how companies can create value in this economic scenario, it is important to understand better the two fundamental factors: big data and machine learning; dispelling some of the clichés that limit their understanding by companies and, therefore, the possibility of extracting value from them.
In the first half of this decade, the term “big data” became popular in the management field. This was first coined in 1997 by two NASA researchers, Michael Cox and Davis Ellsworth. The two researchers, overwhelmed by the difficulty of managing an ever-increasing amount of data generated by their studies, wrote: “A set of generally very large data, such as to put a strain on the capacity of the memory, the hard disk, and even the local disc of a computer. We call this the big data problem.”
In the management field, in 2011, the McKinsey Global Institute defined big data as “datasets whose volume is so large that it exceeds the capabilities of software to capture, store, manage, and analyze”.
What is big data and why is it so important?
In light of these definitions, we are, therefore, often led to identifying the fundamental characteristic of big data in their quantity which is accompanied by a purely technological problem, namely the difficulty of our computers in managing this amount of data. However, this technological problem is certainly not new. Indeed, we could say that it is as old as the invention of the computer. For this reason, reducing big data to a mere technological and dimension problem is at least misleading.
So, what makes big data so special? To understand this, let’s take a closer look at the data that millions of people produce every day on Spotify.
Many of us rightly believe that Spotify has a very thorough understanding of our musical taste. Actually, Spotify knows a lot more: It knows, for example, when we run, when we drive, when we are showering, when we go through sad times or happy times. How do you know? Spotify’s servers are full of “Run, Michael, Run!” playlists, “Driving Songs”, or “Songs to Sing in the Shower”. Most people create playlists with the name of the activity they are engaged in while listening to music. The users themselves say: “I’m running”, “I’m driving”, “I’m taking a shower”.
This is the painstakingly detailed level of knowledge about our daily activities that Spotify has access to (and on which it has built much of its business model). Spotify represents the norm of the data-driven economy. We are surrounded by objects that record whatever activity we do and turn it into data, stored somewhere and ready to be used. The real advantage of big data, therefore, lies not so much in the quantity, but in the ability to provide extremely detailed information about an individual. It is precisely this ability to provide us with detailed information on the lives of billions of people that represents the real advantage of big data. The fundamental change that made it possible to transform simple bytes into big data was the advent and proliferation of social networks, a sociological phenomenon that has forever changed our sense of privacy. It is no coincidence that, almost simultaneously with the growth of social networks, a new term has been coined to describe our society: “sharing economy”. Today, we are willing to share with perfect strangers objects that until recently were absolutely private, almost intimate, such as cars, houses, motorcycles, bicycles. Likewise, we have no qualms about sharing private information about us. The need to share our lives with strangers has meant that hardly anyone finds it strange that every step, every note heard, every thought expressed, leaves the walls of their home to end up in supercomputers all over the world.
Ignoring this change – cultural, not just technological – means having only a partial understanding of the value of big data. These have revolutionized our economy because they have reversed the process of creating value. In the past, it was the company that went to the consumer to understand their needs through surveys or focus groups. Today, it is consumers who directly or indirectly provide businesses with a myriad of information about everything they do or think, every minute of their lives. Not the few consumers who could hardly be interviewed, but millions of people around the world.
How can companies use this new resource?
The answer is called “machine learning”. Creating value with big data: machine learning as a key driver of competitive advantage.
There is still some confusion about big data and machine learning, that pushes to clarify the process and techniques.
The process is divided into two phases: training and prediction. In the computer training phase, two types of input are provided: The data and the answers that we want the machine to learn, which in technical terms are called “labels”. Famous is the case of Target which, in 2012, developed a model to identify pregnant women. Let’s see how.
In the training phase, we focus on a group of customers, some of whom we know are pregnant (because after a few months we observe that they buy diapers or baby food) and others who we know are not. We begin to pass the purchase data of a customer to the machine and ask it to tell us if she is pregnant or not. We also provide the machine with the label, in this case, a binary variable: “yes” (if the customer is really pregnant) or “no” (if she is not). The machine creates a first model and gives an answer (totally random at first). Then compare this answer with the correct one, i.e. the label we provided. If the answer is correct, the computer moves on to the next customer. If the answer is wrong, the computer modifies the model to provide a better answer next time. In order to learn, the machine will have to do this loop thousands, in some cases millions, of times. At each iteration, the machine adjusts its model, until it can provide the correct answer in an adequate number of cases (usually, around 70/80%). At the end of this phase, the Target model had learned that pregnant women have certain distinctive characteristics: They buy 25 products more than other customers, such as cotton buds, natural soaps, and vitamins. At this point, the training phase ends and the computer is ready to use the model it has just learned to analyze the data of new customers, who we do not know if they are pregnant or not. We then move on to the so-called prediction phase, where we will give the computer data of new customers and ask if they are pregnant or not. How does the machine predict? Using the model learned during training.
This case is famous because it was one of the first times in which we have seen big data in action in the management field. Analyzing the data of their loyalty cards, Target realized that women who have just given birth are a particularly loyal segment of consumers (probably because they don’t have much time to find and try new stores). Hence the decision to retain them when they are pregnant by sending promotional coupons.
As you can see, the mechanism by which machines learn is very similar to the way humans learn. However, machines need to be trained on huge data, be it customer purchases, Facebook posts, or Instagram images. This need explains why, although many of the machine learning models were conceptualized years and sometimes decades ago, in the management field they have only recently begun to be talked about.
It should be clear, at this point, why big data is considered the fundamental resource in the so-called data-driven economy. Those who own big data, and are able to analyze it, have a fundamental competitive advantage.
Cover image source: ThisIsEngineering