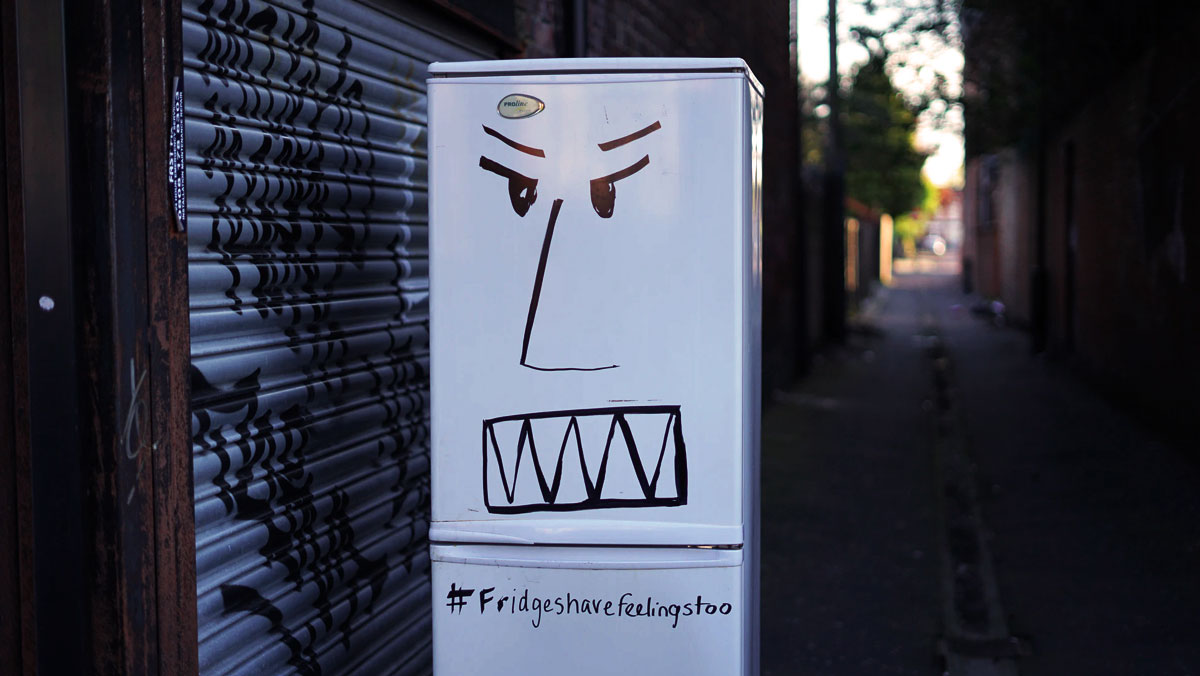
The problem with the written word is that it can often be difficult to gauge the tone — and this is particularly true when it comes to short messages, like texts and social media posts. Often, we fail to identify a tone such as sarcasm or aggression or we assign tones when they were not intended. This can lead to misunderstandings and arguments in personal communications, but in business, it can have devastating results.
For a business, how you are perceived through your social media presence is incredibly important and it’s vital that business owners and managers know what is being said about the brand. ‘Sentiment analysis’ allows businesses to monitor customer chatter on social media in order to get a picture of how the brand is perceived.
Here is an overview of what ‘sentiment analysis’ is and how you can leverage it for your brand:
First things first — What is sentiment analysis?
In a nutshell, sentiment analysis is an algorithm created to ascertain the emotional landscape of a written message in order to grade it positive, negative, or neutral. In some cases, the analysis can assign a more specific emotional reaction such as anger, laughter, or love, and it does it through machine learning or opinion mining. This increasingly-popular tool is becoming so vital that a huge number of companies — and even government agencies — have gotten on board with it.
The above diagrams give you an idea of the kind of results which are netted by sentiment analysis.
How does it work?
We could go into the nitty-gritty of all of the processes, methods, and algorithms which go into making sentiment analysis work but, for now, we’ll keep things simple. To be honest, you don’t really need to know about any of that.
What you do need to know is that there are tools that will handle all the complex bits for you, quickly, reliably, and inexpensively. I personally use Brand24, but there are other tools out there for you to choose from.
What you need to know
- Sentiment analysis is rule-based and, therefore, uses a set of manually-created rules rather than an automatic system. The rule system defines polarity and subjectivity through script language in order to analyze the data and clearly define the parameters;
- It harnesses machine-learning processes to sort through data and then to learn from it in order to accurately and intelligently record its readings;
- It compares and contrasts the rules and machine learning in order to provide a result based on the best of three.
These diagrams show us that, surprisingly, the most negatively-perceived business is an agricultural company rather than a greedy bank or tax-evading corporate giant.
Unfortunately, the machine learning part of the process is not really able to recognize some of the nuances of emotional language such as irony or sarcasm. It is for this reason that the hybrid system is in place in order to combine polarity with more advanced emotional language.
What are the advantages?
- Fully scalable, allowing data to be processed without too much time, hassle or expense;
- Right here, right now — Sentiment analysis works in real time, meaning that you can view customer opinions as they happen, allowing you to react quickly.
Reasons to use sentiment analysis
The ability to quickly and accurately identify negative feedback in order to react in real time and make necessary improvements. Sentiment analysis is able to monitor customer reaction to product changes in order to prevent social media crises. Similarly, it pinpoints positive feedback, allowing you to see what you’re doing right and recruit brand ambassadors.
Recent problems for budget-airline Ryanair have instantly reflected in its sentiment analysis as above.
The above graph shows that, despite some bad press, coffee giant Starbucks is still seen in a positive light.
What scope does sentiment analysis have?
This speaks to the depth of the analysis which, with sentiment analysis, works on three levels: document, sentence, and sub-sentence. These three levels are combined to produce an accurate overview of the emotional landscape.
Fine-Grained
This aspect allows for more detail, for example, very negative rather than just negative;
Emotion Detection
This complex machine-learning-based algorithm works to identify specific emotions in the text, such as anger or happiness.
Aspect-Based
This is, again, a more detailed look — for example, the polarity will be specific, such as ‘There are fewer chocolates in a Quality Street box every year!’ This specifically targets an unwelcome change rather than an overall dissatisfaction with a product.
Intent
This speaks to what the writer wants from their message and helps to figure out whether they are complaining about a product or simply asking for help or assistance regarding a product or service.
Language
This really tricky area can be navigated for businesses with a large international presence but needs to be worked very carefully to avoid misinterpretation and misunderstanding.
How accurate is sentiment analysis?
This is, of course, the million-dollar question. If you’re after an actual figure, then sentiment analysis is estimated to be around 80% accurate most of the time. Which, to be honest, is pretty impressive for such a complex system.
There’s a long way to go before the system is anywhere near 100% accurate. However, it’s a really good tool for businesses who want to know how they’re perceived. The following examples lend a sense of perspective to any accuracy issues:
Intent is something that sentiment analysis seems to particularly have problems with:
The following is another example of how the analysis may fail to see the whole picture as it’s been given a high influencer score and yet has been recorded as negative overall.
The problem here is that the name Uber is mentioned but the context of the message is not actually about the company.
This is a great example of allocating the mention as neutral, which is absolutely correct.
When the negative filter is put in place with the threshold to the max, the above still didn’t reflect a whole picture — purely because the word ‘hate’ was used.
In the above instance, the trigger word was ‘worst’.
What are the main issues?
Sentiment analysis is not perfect and does have the expected quirks and problems. Below are some of the main ones, identified by users:
- Context of language. When sentiment analysis scans a chunk of text, it is essentially interpreting the words without the ability to look into context or backstory — which, of course, can alter the result;
- Sarcastic content and humor. When scanning text containing sarcasm, the tool will often produce an incorrect result due to a misunderstanding of sarcasm;
- Compare and contrast. Analysis can fail when a user is commenting on a change or alteration in a product or service or comparing products from different suppliers. Because this is based on context, it will rarely produce a truly accurate result.
In conclusion
This is a brilliant tool for businesses that are proactive in finding out what their customers are thinking and want to nip any negative publicity in the bud.
Although sentiment analysis has its glitches, you can minimize these by occasionally checking through content to ascertain intent and context. Think of it like a Satnav — you wouldn’t blindly drive into a lake because the machine tells you to; you would figure out that maybe it wasn’t 100% accurate and take evasive action in order to stay on terra firma. As more and more of our lives are conducted on the world wide web, sentiment analysis will become one of the most important tools that your business will use.
Image source: Old Youth